Mastering AI-Powered Data Integration: A Definitive Guide for Success
Artificial Intelligence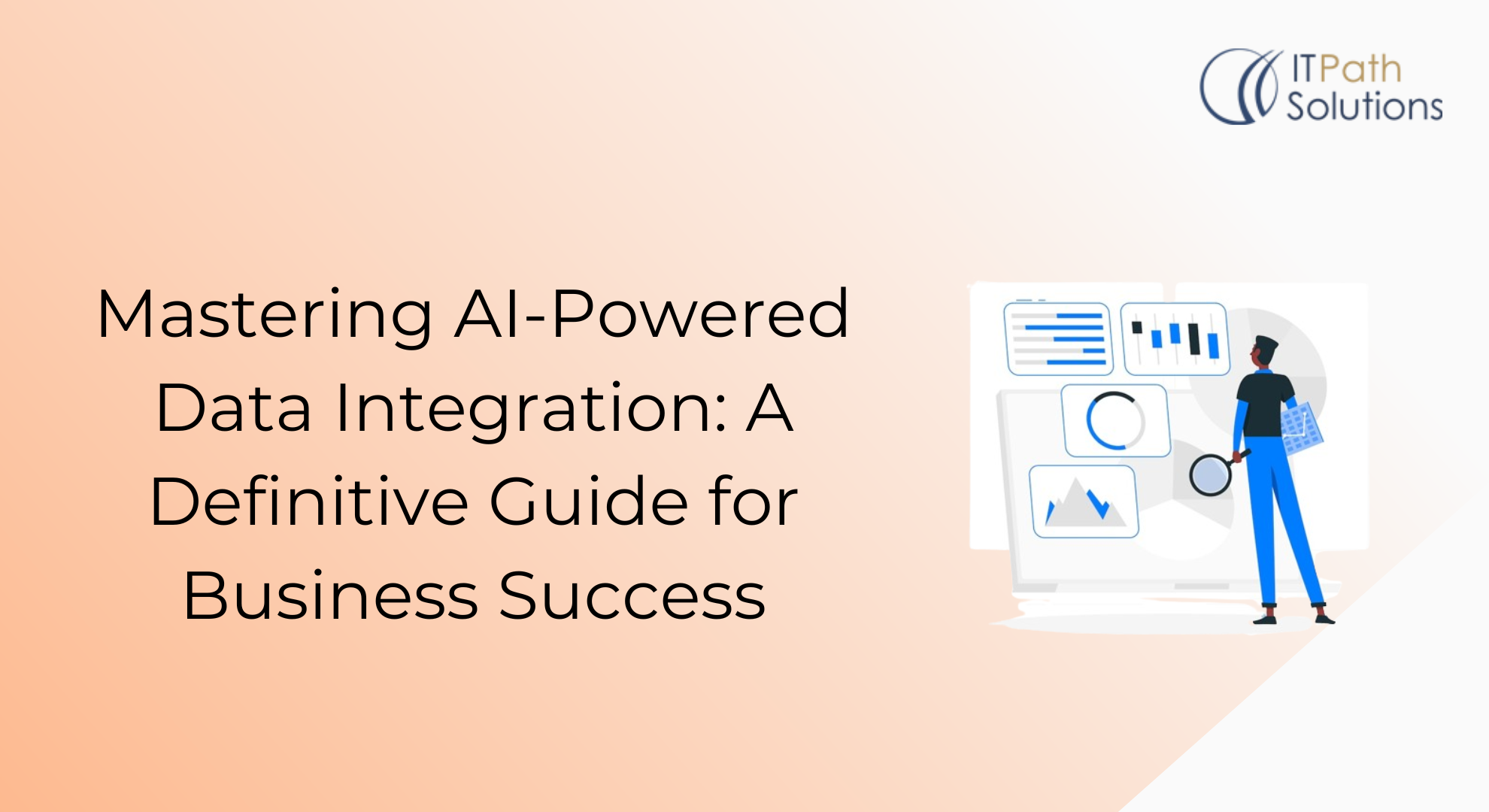
Introduction
Data integration refers to the process of combining data from multiple sources into a unified and coherent view. This unified view enables businesses to gain insights, make informed decisions, and drive strategic initiatives. Data integration involves various techniques and technologies to ensure that data is accurate, consistent, and accessible across different systems and platforms. Your goal should be to go from data integration to consistent and insight worthy data analysis with successful data integration.
Data integration plays a crucial role in modern business operations for several reasons:
- Unified View of Data: By integrating data from disparate sources such as databases, applications, and external sources, organizations can create a single source of truth that provides a comprehensive view of their business activities.
- Improved Decision-Making: Access to integrated data enables stakeholders to analyze information more effectively, identify patterns and trends, and make data-driven decisions that drive business growth and competitiveness.
- Enhanced Efficiency: Data integration streamlines processes by eliminating manual data entry, reducing duplication of effort, and automating repetitive tasks. This efficiency translates into cost savings and improved productivity.
- Better Customer Insights: Integrated data enables organizations to gain a deeper understanding of their customers by consolidating information from various touchpoints such as sales, marketing, and customer service. This insight allows businesses to personalize interactions, improve customer satisfaction, and drive loyalty.
- Agility and Innovation: With integrated data, organizations can respond quickly to changing market conditions, emerging trends, and competitive pressures. This agility enables businesses to innovate, launch new products and services, and capitalize on opportunities more effectively.
Artificial intelligence (AI) technologies are increasingly being used to enhance data integration processes. AI offers several advantages, including:
- Automation: AI-powered tools can automate many aspects of data integration, from data cleansing and transformation to data mapping and reconciliation. This automation reduces manual effort and speeds up the integration process.
- Advanced Analytics: AI algorithms can analyze large volumes of data to uncover insights, patterns, and correlations that may not be apparent through traditional analysis methods. These insights can inform data integration strategies and drive business outcomes.
- Predictive Capabilities: AI models can predict future trends, behaviors, and outcomes based on historical data, helping organizations anticipate changes and make proactive decisions.
- Natural Language Processing (NLP): NLP technology enables AI systems to understand and interpret human language, making it easier for users to interact with data integration tools through voice commands or written instructions.
- Machine Learning: Machine learning algorithms can continuously learn from data to improve data integration processes, detect anomalies, and optimize performance over time.
Understanding AI-Powered Data Integration
AI enhances traditional data integration processes by introducing automation, intelligence, and adaptability into the process. Unlike traditional methods that rely on manual coding and rule-based approaches, AI-powered data integration systems can learn from data, adapt to changing conditions, and improve over time. This enables organizations to streamline and accelerate data integration tasks, reduce errors, and enhance the quality of integrated data.
Benefits of Using AI for Data Integration:
- Automation: AI automates repetitive tasks such as data cleansing, transformation, and mapping, reducing the need for manual intervention and speeding up the integration process.
- Efficiency: AI-powered data integration systems can process large volumes of data quickly and accurately, enabling organizations to integrate data from multiple sources in real-time or near-real-time.
- Accuracy: AI algorithms can detect patterns, anomalies, and inconsistencies in data, improving the accuracy and reliability of integrated data. This ensures that decision-makers have access to high-quality information for analysis and decision-making.
- Adaptability: AI systems can adapt to changes in data sources, formats, and business requirements, making them more flexible and resilient in dynamic environments. This enables organizations to respond quickly to new opportunities or challenges without disrupting existing integration processes.
AI can help you speed up your data integration & analysis
Overview of AI Techniques Used in Data Integration
Machine Learning (ML): ML algorithms can learn from data to identify patterns, relationships, and trends, enabling predictive modeling, clustering, and classification tasks in data integration.
- Natural Language Processing (NLP): NLP technology enables AI systems to understand and interpret human language, making it easier for users to interact with data integration tools through voice commands, text inputs, or conversational interfaces.
- Predictive Analytics: Predictive analytics algorithms use historical data to forecast future trends, behaviors, and outcomes, helping organizations anticipate changes and make proactive decisions in data integration processes.
- Deep Learning: Deep learning algorithms, such as neural networks, can analyze large volumes of unstructured data, such as images, text, and audio, to extract insights and patterns for integration into structured data sources.
- Reinforcement Learning: Reinforcement learning algorithms can optimize data integration processes by continuously learning from feedback and adjusting their actions to maximize performance and efficiency.
By leveraging these AI techniques, organizations can unlock new possibilities in data integration, from automating routine tasks to uncovering hidden insights and improving decision-making capabilities. AI-powered data integration is poised to revolutionize how organizations manage and leverage their data assets, driving innovation, competitiveness, and growth in the digital age.
Key Considerations for Implementing AI-Powered Data Integration
- Data Quality and Preprocessing: Ensuring data accuracy, completeness, and consistency before integration is crucial for the success of AI-powered data integration projects. Organizations must invest in data quality tools and processes to clean, validate, and standardize data from different sources. This includes identifying and addressing issues such as missing values, duplicates, outliers, and inconsistencies to ensure that integrated data meets quality standards and provides reliable insights for decision-making.
- Security and Privacy: Addressing concerns related to data security and privacy is paramount when implementing AI-powered data integration solutions. Organizations must implement robust security measures to protect sensitive data from unauthorized access, breaches, and cyber threats. This includes encrypting data during transmission and storage, implementing access controls and authentication mechanisms, and complying with regulations such as GDPR, HIPAA, and CCPA to safeguard customer privacy and maintain regulatory compliance.
- Scalability and Flexibility: Designing systems that can handle large volumes of data and adapt to changing business needs is essential for successful AI-powered data integration initiatives. Organizations must invest in scalable infrastructure, such as cloud-based platforms and distributed computing technologies, to process and analyze data efficiently. Additionally, they should design flexible architectures and workflows that can accommodate new data sources, formats, and processing requirements as business requirements evolve.
- Integration with Existing Systems: Seamless integration with existing IT infrastructure and applications is critical for maximizing the value of AI-powered data integration solutions. Organizations must ensure interoperability and compatibility between data integration tools and existing systems such as databases, enterprise applications, and analytics platforms. This includes implementing standards-based integration protocols, APIs, and connectors to facilitate data exchange and workflow automation across different systems and platforms.
- By addressing these key considerations, organizations can overcome challenges and maximize the benefits of AI-powered data integration, enabling them to unlock the full potential of their data assets and drive innovation, competitiveness, and growth in the digital age.
Use Cases and Examples
- 1. Customer Relationship Management (CRM): Utilizing AI to integrate customer data from multiple sources enhances CRM capabilities for personalized marketing and sales efforts. AI-powered data integration enables the consolidation of customer information from various touchpoints, including social media, transaction history, customer support interactions, and website behavior. This unified view allows organizations to create detailed customer profiles, predict customer preferences, and tailor marketing campaigns and sales strategies for individual customers. The result is a more personalized and targeted approach, leading to increased customer satisfaction, loyalty, and revenue.
- 2. Supply Chain Management: Leveraging AI-powered data integration in supply chain management optimizes inventory management, forecasting, and logistics. By integrating data from suppliers, manufacturers, distributors, and other stakeholders, organizations can create a comprehensive and real-time view of their supply chain. AI algorithms analyze historical data, demand patterns, and external factors to improve demand forecasting accuracy, leading to better inventory planning and reduced stockouts or overstock situations. Additionally, AI helps optimize logistics operations by predicting delivery times, identifying potential bottlenecks, and enhancing overall supply chain efficiency.
- 3. Business Intelligence and Analytics: Integrating data from disparate sources is a fundamental use case for AI-powered data integration in business intelligence and analytics. By aggregating data from internal databases, external sources, and various applications, organizations can create a unified data warehouse that serves as the foundation for robust analytics. AI techniques, such as machine learning and predictive analytics, enable organizations to extract actionable insights, identify trends, and make informed, data-driven decisions. This integrated approach empowers businesses to gain a holistic view of their operations, customer behavior, and market trends, driving strategic planning and competitive advantage.
- These use cases exemplify how AI-powered data integration transforms business processes across different domains, offering enhanced capabilities and strategic advantages. As organizations continue to explore innovative ways to leverage their data, AI-powered integration becomes a pivotal enabler for achieving efficiency, agility, and intelligence in decision-making.
Best Practices for Successful AI-Powered Data Integration
- Define clear objectives and requirements: Establishing goals and criteria for data integration projects
- Collaborate across teams: Involving stakeholders from IT, business, and data science teams to ensure alignment and collaboration
- Test and iterate: Conducting thorough testing and validation to identify and address issues early in the process
- Monitor and optimize: Continuously monitoring performance and refining AI models to improve accuracy and efficiency
Conclusion
In conclusion, AI-powered data integration holds immense potential for revolutionizing business operations across various domains. By seamlessly integrating data from multiple sources, organizations can unlock valuable insights, enhance decision-making capabilities, and drive innovation and competitiveness. From customer relationship management to supply chain optimization and business intelligence, AI-powered integration offers a powerful toolkit for extracting maximum value from data assets. As organizations continue to invest in AI technologies and embrace data-driven strategies, the importance of effective data integration will only grow, paving the way for a future of unprecedented efficiency, agility, and growth in the digital age.