Self-Service Analytics: Strategies & Best Practices
Power BI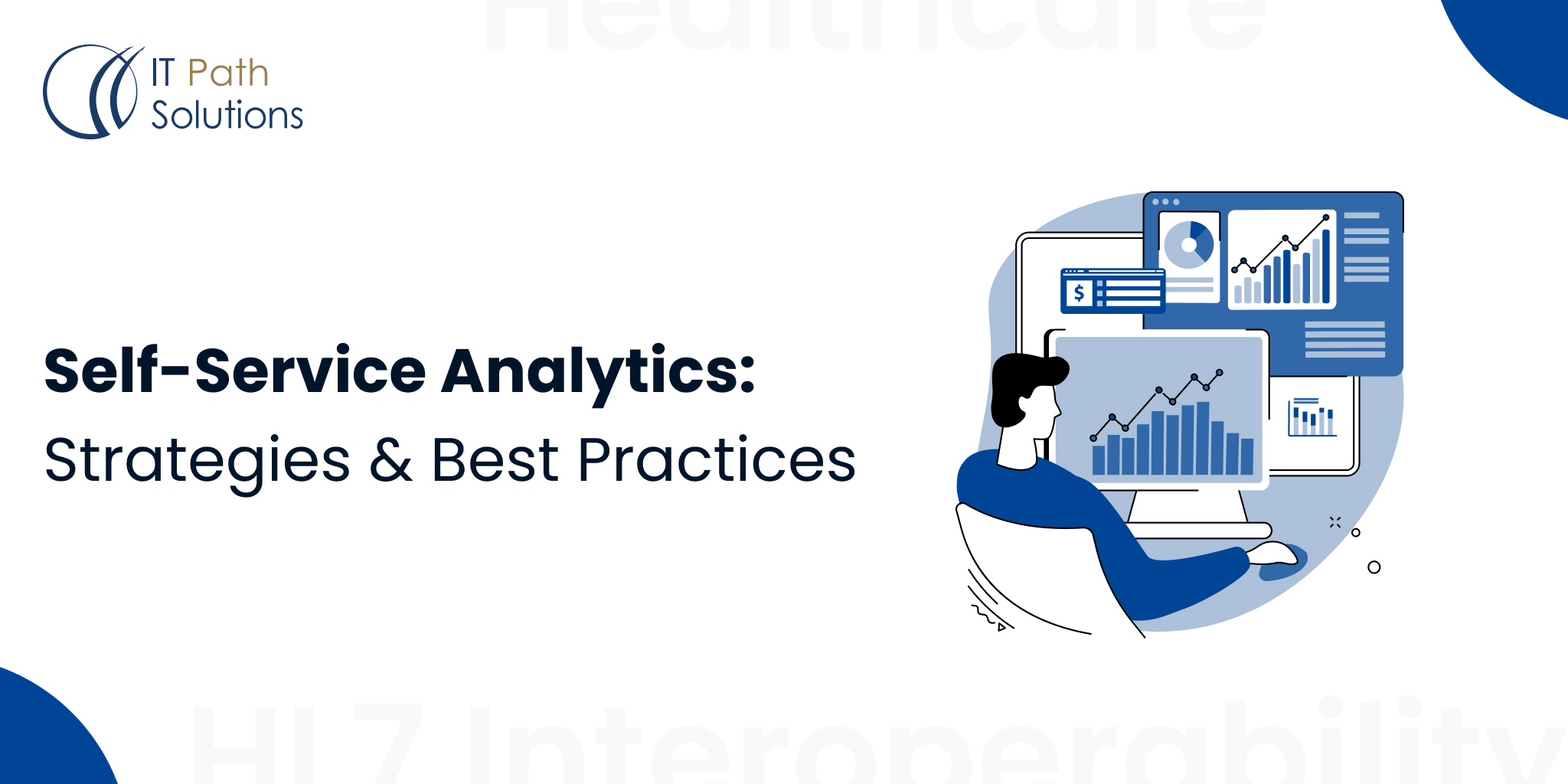
What is Self-Service Analytics?
You know that old trope about data analysts holding the keys to all your company’s juicy insights? Self-service analytics is basically giving everyone a copy of those keys. It’s the cool new approach of putting data exploration and analysis powers directly into the hands of business folks across teams like marketing, sales, and operations. No more pestering the data nerds for every little report!
Traditionally, data scientists and analysts were the gatekeepers. They gathered requirements, prepped datasets, ran analyses, and dolled out insights like prized possessions. But as companies have become more data-obsessed, that bottleneck just doesn’t cut it anymore. Self-service data analytics blows the gates wide open with user-friendly tools that abstract away all the technical complexities.
With these self-service platforms, even non-technical users can connect data sources, explore visually, build reports and dashboards, run ad-hoc analyses — you know, the whole nine yards. They tap into slick data viz, natural language querying, automated modeling, machine learning…all that good stuff to make analysis super accessible.
The core idea is empowering a broader crew to derive insights themselves, while leaving the real gurus free to focus on advanced data witchcraft. It’s about fostering that glorious data-driven culture across teams.
What makes it truly ‘self-service’?
For an analytics tool to be worthy of the self-service badge, it needs certain hallmarks that empower us regular business folk:
Intuitive UI/UX: We’re talking visually appealing dashboards, drag-and-drop interfaces, and natural language search — basically, an experience designed to abstract away the usual technical clutter and jargon. Even a social media manager should be able to navigate this seamlessly.
Simple Data Access: Connecting and blending data from multiple sources can’t be a soul-crushing experience. The best self-service platforms have pre-built connectors to common data repos and file formats. No more bugging IT to grant us access to that server.
Automated Data Prep: Let’s be real, the most soul-crushing part of data work is prepping datasets — cleaning, transforming, and enriching. Self-service solutions use AI/ML to automate painful tasks like profiling, schema mapping, and join detection. More insights, less grunt work.
Rich Data Visualization: Impactful Data Visualization is the bread and butter of self-service. We need customizable charts, graphs, maps…basically every flavor of visualization to explore datasets from all angles.
Ad-Hoc Analysis: A huge win of self-service is being able to analyze data spontaneously without rigid processes or writing scripts. Slice, dice, drill down, build calcs on the fly to chase those fascinating insights.
Collaboration/Sharing: Since these insights are meant for the masses, collaboration features that enable sharing, annotating, and advancing analyses further as a team are vital.
Why Self-Service Analytics Matters
Look, companies can’t afford to treat their data like a precious gemstone locked away from the common folk. Self-service is about democratizing those analytical powers across the entire organization to unlock maximum value from data investments.
It empowers decision-makers in every department to drive insights independently without overwhelming those analyst teams constantly. That accelerates the speed at which new discoveries can fuel actions and strategies, allowing businesses to be far more agile in responding to market shifts, customer demands, and new opportunities — you get the drift.
But beyond just operational agility, self-service amplifies ROI from your data infrastructure, integration pipelines, governance…the whole nine yards. With more folks extracting insights, those investments get maximized instead of insights being siloed with limited usability.
Self-service also unleashes the full analytical potential of employees across roles. They get to pursue new inquiries and derive value rather than waiting around for static reports from the analysts. That autonomy sparks innovation as teams can basically crowdsource insights and rapidly validate hypotheses through hands-on data experimentation.
Collectively, self-service analytics equips businesses with the crucial speed, widespread data literacy, and innovative data culture needed to thrive in our uber-competitive, data-driven world. Those are some legit competitive advantages, friend.
What are the business benefits?
- Faster time to insight by cutting the analyst dependency
- Improved data-driven decision-making org-wide, not just certain teams
- Enhanced business agility to sense and respond to changes rapidly
- Increased ROI by maximizing the usability of your data investments
- Higher employee productivity and value generation from democratized insights
Best Practices for Self-Service Analytics
Okay, I get it; you’re sold on this self-service revolution. But how do you adopt it without getting burned? Embedding self-service analytics within an organization is not simply the purchasing of a cloud-based tool and the turning on of a hypothetical “on” switch. This approach will be considered when rolling it out in the enterprise concerning business strategy and technology considerations, which will be brought to bear, provided it may focus on aspects such as training workers and putting in place data standards. Below are some fundamental best practices and strategies in the effective roll-out of self-service analytics in an organization.
Check your needs.
Take a good look before you leap into self-service analytics—at the teams, areas, and projects that would make the most of it. Select the areas and projects where making quick, data-driven decisions could most assist the business. Starting with key, high-value projects allows you to show off some early wins and makes it a lot easier to get more support later.
Source for the enabling platform provider
As you choose your self-service analytics platform, look at how easy it is to use, how well it works, if it can connect with other tools, if it can grow with you, and if the provider is reliable. The key is to find the right platform, one that fits what your group needs. Again, look at a big picture that considers factors like the skill levels of intended users and the platform’s ability to fit in with your other systems and data sources.
Automated data pipelines
This platform is focused on self-service analytics platforms that are easy to use through intelligent technology, simplifying data setup tasks such as sorting data, performing tasks on data layouts, connecting data parts to others, and changing data where needed. These automatic data flows make getting insights quicker while keeping data right and in line across the company.
Begin with the quick wins.
Above all, place quick wins first. You don’t need to start with everything; pick a few critical issues that self-service analytics can quickly resolve to score wins for the business. You show how useful self-service analytics are to clear problems or urgent needs to give confidence in the solution and, thus, a strong use case to justify further investment and expansion.
Elaborate on something more forward-thinking
After simple self-service data efforts succeed and mature, extend these tools to larger groups and gradually take on more challenging problems. Drill into future trends, conduct live data checks, and bring in external data that may expose deeper, brighter insights.
Encourage People
Advocate for trials and tests. In a business, inculcate a culture that is in love with data by pressing teams to try self-help data tools, to look into the data, to ask new questions, and to do different checks. This love for tests democratically brings the newest of these ideas, which ways usually may miss important stuff.
Data Governance
Develop and operationalize strong data governance policies and procedures, including but not limited to access controls, data standardization, security, and privacy, as self-service analytics continue to take hold. Put clear guidelines and processes in place to ensure that there are enough guardrails around the work when dealing with an increasing number of users who are interacting with data.
Identify data gaps
Analyze all data assets available in the organization and identify some of the most critical gaps that greatly hinder the full adoption of self-service analytics. Fill in with data the most critical sets or sources needed for answering business-critical questions, and therefore, with a very high business value because any missing fields will discourage any potential insights.
Develop data readiness
Data Prep—Make data ready. Ensure that your self-service platform is built with easily obtainable data connectors, allowing access to clean, well-formatted, and analysis-ready sources of information. This also involves some preprocessing and cleansing of data, data transformation, and data enrichment projects, getting datasets to a state where they can be easily consumed on a self-service platform.
I am very excited about implementing an infrastructural and architectural data setup that is scalable enough to cater to many more self-service analytics use cases when the organization develops and adopts them at scale. This spans from storage and computing power to data engineering resources.
Establish organizational data standards
Implement a scalable data infrastructure and architecture that can handle increased analytics workloads due to self-service use cases and adoption in the organization. It should cover storage, computing power, and data engineering resources.
Setting up organizational standards for data Define and enforce clear standards for data management, naming conventions, formatting, and metadata to ensure consistency and integrity are preserved as more users interact with data through self-service analytics.
Prioritize data security, privacy, and compliance
Since self-service analytics broadens access to data for a large audience, sensitive data should be protected with due care. Implement strict access controls, data masking, and audibility in self-service so that unauthorized access or misuse is impossible and compliance with applicable regulations can be ensured.
Be mindful of self-service analytics limitations
Even though self-service analytics can provide many benefits in most use cases, the very advanced and highly specialized analyses will still be left up to the skills of data professionals. It provides a level playing field for all to democratize data but will still leverage analysts to the maximum extent possible.
Challenges and Solutions in Self-Service Analytics
In reality, the search for self-service analytics brings in both the challenges and the solutions. Therefore, even as it has its benefits, several challenges are bound to stand in the way of businesses realizing value from this approach. Overcoming these challenges and guaranteeing the full acceptance of self-service data analysis is pegged on sufficient planning, implementation, and continuous governance. Some of the challenges are outlined together with their solutions below:
- Data: containing inconsistencies may bring some insights. Use data quality tools and processes to make your data sources still hold.
- Data security: The more data expands its reach, the more the security risks escalate. Implement masking, access controls, and auditing capabilities.
- Collaboration and Communication; The silos created by self-service run the risk of making little headway to the failure of users to share their findings. Enable sharing and collaboration but within platforms.
- Low data culture and literacy: Lack of understanding may be a barrier to usage of self-service analytics tools. Training programs are there. Inculcate a mindset in which data-driven decision-making is valued.
Also Read: Self-Service Analytics: Key Checklist Before Embedding
Our Method to Infuse Self-service Analytics
Therefore, our approach to self-service analytics embedding ensures successful, sustainable adoption that fits the uniqueness of the needs and requirements of each organization. The process begins with an exhaustive assessment: means of the data environment, processes, and level of data literacy to devise a unified data strategy for the harmonization of self-service with centralized governance.
We are selective in the choice of self-service platforms and complementary tools according to functional requirements, the level of skills, and expectations of scalability. We know that self-service is a considerable transformation, so our change enablement programs will prepare the organization for long-term adoption. The first step is deployment. This is a continual program of optimization: self-service analytics are monitored in real-time, governance adjustments are made by adding new sources, and enhancement of capabilities enhances lockstep with changing business needs.
This is where the process of self-service embedding—in the case of IT Path—goes one step over deploying tools: at the core of optimization changes to a data-driven operating model that drives better value from your information asset of business intelligence solutions
FAQs
Q: Who can use self-service analytics tools
A: Self-service analytics democratizes data and makes insights more accessible to all business roles, from marketing and sales to nce and operations.
Q: Are data analysts still in market demand in the age of self-service analytics?
Most of the analytics will be done in self-service ways, but of course, skilled data pros will be needed for the higher end of the spectrum: modeling, data engineering, and data strategy.
Q: Which skills are demanded for self-service analytics?
A: Yes, it will be self-service for non-technical users, but some knowledge of data literacy would be helpful to analyze it comprehensively.
Sign out time!
Self-service analytics changes the game’s rules, putting power with rich analytical, and organizational capabilities in the hands of business decision-makers. Organizations can democratize data today, achieving maximum benefits through the right strategies and best practices for developing a data-driven approach.